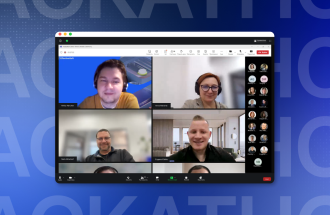
Contact us
Our team would love to hear from you.
The use cases of ML make this technology a critical part of digital transformation. This article explains how ML can be implemented in the supply chain and logistics, its importance and benefits, as well as the challenges ML can help overcome.
ML is a branch of AI that imitates human learning activity using data and algorithms. This technology has already demonstrated its value across various industries, enabling businesses to adapt to a new world and achieve success. ML has the potential to revolutionize the supply chain and logistics, boosting efficiency and promoting sustainability.
Effective supply chain and logistics management requires detailed planning, skillful implementation, and thorough control of the flow of goods and services. ML optimizes and automates supply chain these processes, allowing companies to enhance decision-making with valuable insights from data produced by ML algorithms and helping them build resilient supply chains.
The global generative AI market for the supply chain sector is projected to reach almost $13 billion by 2032, with the logistics sector expected to approach $19 billion.
By the end of 2024, 50% of supply chain companies will have invested in applications that support AI and advanced analytics capabilities.
AI-based approaches to forecasting reduce forecasting errors by up to 50%.
AI-enabled supply chain management allowed early adopters to improve logistics costs by 15%, inventory levels by 35%, and service levels by 65%.
AI adoption rates in supply chain and manufacturing businesses worldwide in 2022 and 2025.
The global market size of ML is estimated to surpass $771.38 billion by 2032.
In the current environment, supply chain and logistics businesses face challenges that can impact their prosperity and compromise their market position. ML can help them persevere through hardships and outperform competitors.
Logistics and supply chains face many potential risks that can impact businesses—market changes, reliance on suppliers and other third parties, transport availability, unexpected delays, supply chain disruptions, consumer demand, and even political agendas. A comprehensive risk management strategy should consider all the variables affecting the flow of goods. Integrating machine learning in logistics and supply chain management facilitates well-informed and rapid responses to any unexpected events. ML algorithms provide insights based on unseen data patterns and trends that are difficult to identify otherwise, help forecast complex scenarios, and enhance the decision-making process.
An efficient supply chain requires effective planning and the optimized allocation of workforce, inventory, vehicles, and other resources. Interruptions and inefficient resource usage lead to increased costs, low product quality, and customer dissatisfaction. ML-enabled solutions help companies base their resource management decisions on accurate real-time and historical data from various sources.
Global logistics and supply chain operations are heavily impacted by the geopolitical situation. Sanctions, protectionism, trade wars, reputational risks, and major global events all affect the cost, availability, and reliability of goods. ML models help build resilience toward supply chain disruptions by tracking and analyzing data, forecasting, modeling risks and scenarios, and providing solutions in real time.
MGLogistic simplifies KPI monitoring (e.g., orders, shipping times, customer satisfaction, transportation costs) and streamlines logistics systems (orders, billing, customer relations). Enables in-depth trend analysis for quick, informed responses.
The adoption of ML algorithms in the supply chain and logistics industries is part of digital transformation, an integral process for companies looking to stay ahead of competitors and provide customers with top-notch services. Businesses are increasingly recognizing the need to integrate ML into their processes due to the significant advantage this technology provides. Following are some of the important benefits ML can deliver.
ML algorithms process large data volumes from all relevant sources to provide companies with actionable insights to inform decision-making. The models are continuously improved through learning and retraining, enabling decision-makers to transform valuable information into effective strategies.
ML facilitates the optimization of the physical flow, including warehouse processes, defect detection, route optimization, inventory management, and other manual tasks. This increases workflow efficiency and reduces the time required for task completion, streamlining supply chain and logistics processes.
With the help of ML models, data is tracked through all supply chain stages, from raw materials to the delivery of a product to a consumer. End-to-end monitoring allows companies to see problems in the workflow and develop solutions, as well as predict and reduce risks and build trust-based relationships on all levels of the supply chain.
Machine learning in the retail supply chain allows businesses to predict customer needs and demands and provide them with personalized services. Optimized routes and streamlined processes not only contribute to operational efficiency but also enhance customer satisfaction by reducing delivery time and minimizing the likelihood of shipping errors or faulty products.
Most use cases of ML models in supply chain and logistics help companies significantly reduce waste and increase sustainability. Route optimization reduces fuel consumption and carbon emissions; accurate demand forecasting reduces unnecessary product manufacturing; and efficient warehouse management promotes eco-friendly energy consumption.
The application of machine learning in logistics and supply chain can maximize productivity, accuracy, and efficiency.
Accurate and reliable demand forecasting is one of the most significant use cases of machine learning in supply chain as it helps businesses revise resource usage, prevent under or overstocking, quickly adapt to dynamic market conditions, and effectively respond to any changes or threats. ML models analyze various factors that impact future demand, including historical data, market and industry trends, and customer behavior.
Effective inventory management can be achieved using ML algorithms, enhancing visibility and minimizing human error. ML-powered inventory management systems provide businesses with detailed real-time data about each product and its performance, inventory levels, and more, while simultaneously providing customers with information about the availability of specific items.
Efficient warehouse storage and product handling minimizes waste, improves resource allocation, and reduces order fulfillment time. With ML algorithms, businesses can control order picking, packing, and shipment; optimize space usage and layout; and monitor operational performance.
A notable use case of machine learning in logistics is route optimization. ML models analyze different factors and dynamic variables, such as weather conditions, traffic, and road works, to develop the most efficient routes and adjust them in real time when necessary. This enables companies to lower costs by reducing distances and optimizing fuel usage, which enhances operational efficiency, improves customer service, and facilitates on-time product delivery.
Price optimization strategy gives companies a competitive advantage and improves customer satisfaction and loyalty. By analyzing market conditions and other factors that influence prices, such as customer behavior and preferences, supply, and competitors’ prices, ML algorithms help enhance decision-making and make pricing policies more dynamic and adaptable.
One of the most crucial elements of any supply chain is the financial transactions between suppliers, retailers, and customers. With supervised learning, an ML model can be trained to accurately identify abnormal transactions in payment systems, enabling further investigation to prevent credential abuse and mitigate security breaches.
Vehicles and equipment require constant monitoring, and ML can automate this process. Using ML algorithms, businesses can predict maintenance needs based on data from sensors, usage patterns, and other conditions. This helps avoid unexpected expenses for repairs, reducing maintenance costs.
When it comes to package delivery, ML-powered drones and self-driving cars are examples of how machine learning in the logistics industry can revolutionize processes. These solutions decrease delivery time, enhance customer satisfaction, improve accuracy, and significantly reduce expenses. For example, operational costs for autonomous delivery drones are 70% lower than regular van delivery.
A product goes through multiple stages in the supply chain before it reaches a customer. Initially, manufacturers strive to ensure products are free from defects. However, packages and goods may sustain damage during transportation. ML-powered automated quality inspection at each stage identifies flawed or low-quality products, thereby minimizing errors and reducing the occurrence of faulty goods received by consumers.
A supply chain is a complex system, with successful operation determined by numerous factors. ML is an innovative technology in this field that enables businesses to overcome challenges and increase productivity with powerful use cases. To learn more about how ML can improve supply chain processes, contact the ML experts at EffectiveSoft. Our team will help you identify opportunities for improvement and design an ML-powered solution that precisely matches your needs.
Our team would love to hear from you.
Fill out the form, and we’ve got you covered.
What happens next?