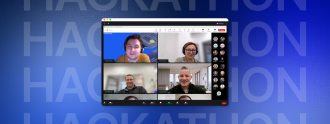
Contact us
Our team would love to hear from you.
Over the past few years, artificial intelligence (AI) and its subsets have become indispensable tools in the financial industry. With machine learning algorithms and neural networks, subcategories of AI, companies can predict market trends and make well-informed decisions. However, beyond the wealth of benefits, there are certain limitations that financial organizations face when implementing ML-based solutions. The benefits, challenges, use cases, and real-life examples of ML in finance are detailed in this article.
Machine learning is a subset of AI designed for machines to learn, draw insights, and make predictions. The magic of ML-powered solutions is that they do not need to be explicitly programmed. Fed with data, ML models automatically adjust their parameters. To keep ML solutions up-to-date and maintain their efficiency, it is preferable for data scientists to retrain the models as frequently as needed.
At the heart of machine learning are algorithms that can process input data to make predictions and decisions using statistical analysis. The more data is contained and the higher its quality, the more accurate the results.
The use of AI and machine learning in finance is steadily evolving. This evolution is easy to explain due to the ability of machine learning systems to process massive volumes of data to uncover patterns within them.
We have listed the topmost machine learning applications in finance to help you understand how financial institutions thrive by implementing ML-powered solutions.
Precise stock market forecasting is hardly possible due to its volatile and non-linear nature. However, it is more feasible using machine learning in trading and finance.
Based on past experiences, ML considers multiple parameters and identifies patterns to show how the market will behave in the future, thereby providing forecasts of future stock prices with high accuracy.
A potential borrower’s creditworthiness is one of the critical factors a lender considers when issuing a loan and agreeing on its terms and conditions. Many banks apply credit and other scoring models that help assess a borrower’s reliability based on statistical methods. Machine learning significantly automates these processes and helps achieve higher accuracy.
Fraud detection has always been a serious problem in banking, healthcare, and other industries that deal with large amounts of money or personal information. The sheer volume of sensitive data makes the financial sector vulnerable and subject to data breaches.
Machine learning offers an advanced and accurate approach to detecting fraud. It identifies patterns that can indicate fraudulent activities, such as unauthorized transactions or a suspicious behavior pattern, which may not be apparent using traditional rule-based systems.
Process automation is among the most common and effective machine learning applications in all industries. ML-powered solutions enable financial service providers to entirely replace manual and Excel work by automating routine tasks to boost business productivity.
Robo-advisors, call center and paperwork automation, gamification of employee training, and chatbots are examples of such automation, which allows financial organizations to replace repetitive manual work, enhance the customer experience, and scale up their services.
The high level of customer experience is the core indicator of the quality of the financial services provided. Companies embrace machine learning finance applications to improve this marker by optimizing services and budgets. These applications help anticipate customers’ needs, provide instant support, personalized recommendations, and valuable advice, as well as resolve basic queries. This ML use case is especially worthwhile for organizations with a large client base.
Customer churn forecasting is about detecting those who are likely to leave or cancel their regular subscription to your services. These metrics are crucial for businesses since the loss of customer loyalty often leads to monetary losses.
Machine learning enables financial companies to predict this churn by analyzing customers’ behavior, preferences, and common reasons for unsubscribing. These predictions give valuable insight into the marketing efforts an organization can make to retain its clients.
Creating and managing a financial portfolio is much easier and smarter with ML-powered chatbot assistants. These solutions use algorithms to oversee and optimize assets aligned with the client’s risk preferences and desired goals. Chatbot assistants help clients handle their portfolios by providing valuable investment advice.
Having reviewed the main machine learning use cases in finance, we can summarize its main benefits. ML-driven solutions allow financial service providers to:
In finance, even a small error can have severe consequences and lead to considerable monetary and reputational losses. With machine learning, the risk of human error is much lower due to its accurate results and ability to detect fallacies in data-sets that humans might miss.
Machine learning algorithms can sniff out the patterns of fraudulent transactions, money laundering, suspicious user access, policy violations, and more, helping financial organizations take steps for their systems’ safety while staying compliant with all regulatory requirements.
By automating the entire process of data analysis through experience-based learning, machine learning systems can reduce workload and optimize workflow, thereby increasing efficiency and productivity.
The ability of machine learning algorithms to detect patterns and make predictions based on historical data can increase the accuracy of decisions by providing data-driven insights.
Losing customers is expensive and benefits competitors. However, the ones who receive quality services, fulfill their demands through these services, and feel cared for are unlikely to leave or change the financial service provider. Machine learning is a great tool for financial companies to address these needs.
By automating processes, increasing staff productivity, enhancing decision-making, predicting market trends, and improving customer relationships, machine learning adoption can result in significant cost savings and revenue growth for businesses.
However, it is not all smooth sailing. To fully enjoy the exceptional opportunities that come with implementing machine learning into company processes, financial organizations have some challenges to overcome. Below, we outline the main ones.
High-quality data is pivotal for machine learning to deliver efficiency. Any issues in this regard can affect the accuracy, timeliness, or consistency of the results, leading to incorrect assumptions and significant losses.
These problems can stem from errors in data collection, processing, or storage, as well as from changes in data sources or formats caused by updates, migrations, or other modifications. The best way to avoid these roadblocks is to perform regular data quality checks throughout the data lifecycle, which imply the validation, cleaning, and documentation of data.
Data availability is often interrelated with data quality challenges. Indeed, sometimes, they can conflict. Thus, improving data quality can reduce its availability, and vice versa.
However, these two concepts have different origins. Usually, data availability issues arise from limitations in data sources or regulations, as well as from failures in data delivery, storage, or transmission systems.
To prevent undercutting in your data use, the best approach is to ensure that multiple data sources are available. In this way, a failure in one server, disk, or other source containing your data will not hinder the proper functioning of your ML system.
Financial data is extremely sensitive because it includes personal data in addition to monetary information, such as bank account information, credit card numbers, investment portfolios, and tax returns. The leakage of such information can result in customers losing substantial sums of money and businesses losing customer loyalty and customers themselves, not to mention years of legal proceedings. However, by processing large amounts of data, ML algorithms can inadvertently reveal confidential information. To avoid this, companies must maximize security by using reliable and unbiased data to train ML models, ensuring compliance with numerous data privacy regulations, and conducting regular audits of their ML models. To ensure their ML systems are safeguarded in the best possible way, they can reach out to an experienced data scientist.
One of the biggest concerns is the shortage of talented data scientists—professionals who can apply large data sets to solve problems through machine learning. These are the magicians who feed machines, helping them make right decisions and take smart actions in real time. If you are looking for a top-notch expert in data science, we are here to help you.
JP Morgan Chase & Co. is one of the biggest American financial services firms. To establish leadership in the financial sector, the company started embracing advanced technologies, including machine learning. Using a proprietary unsupervised ML algorithm for credit scoring, loan underwriting, and more, the bank created efficiencies, reduced man-hours, and served more clients with greater effectiveness, speed, depth, and sophistication.
5Point is one of the largest independent financial institutions in Southeast Texas. Aiming to gain a competitive advantage, increase their loan book, and serve more clients, the company decided to embrace AI tools, including machine learning. With tailored ML models, 5Point can now identify overlooked but creditworthy applicants and get an accurate picture of borrowers’ delinquency risk while reducing risk and improving the bottom line.
Danske Bank is one of the leaders in the Nordic banking system. Headquartered in Denmark, Danske Bank has its core market in Finland, Norway, and Sweden, in addition to its home country.
Since most transactions take place digitally via mobile phones, tablets, and other devices, there is a significant area for fraud to occur. Old methods, such as human-written rules engines, produce insufficient results. For this reason, the company turned to ML-powered solutions.
Implementing machine learning algorithms brought several benefits, such as improved probability predictions, a much higher percentage of detected fraud cases, and reduced false alarms.
ML-powered software is rapidly becoming critical for investors, traders, bankers, and financial experts. With its ability to process large volumes of data, identify hidden patterns, and automate processes, machine learning, along with other digital technologies in the financial industry, can significantly improve the quality of decision-making, increase operational efficiency, and generate revenue.
However, financial services providers must be aware of the potential risks associated with using these technologies and the importance of having fundamental knowledge and skills in place. Do you have an idea for a machine learning solution? Contact EffectiveSoft. Our ML team with strong expertise and an up-to-date skillset is happy to help you!
Our team would love to hear from you.
Fill out the form to receive a consultation and explore how we can assist you and your business.
What happens next?